Datasets & Software
Download PdfLFuji-air dataset: Annotated 3D LiDAR point clouds of Fuji apple trees for fruit detection scanned under different forced air flow conditions
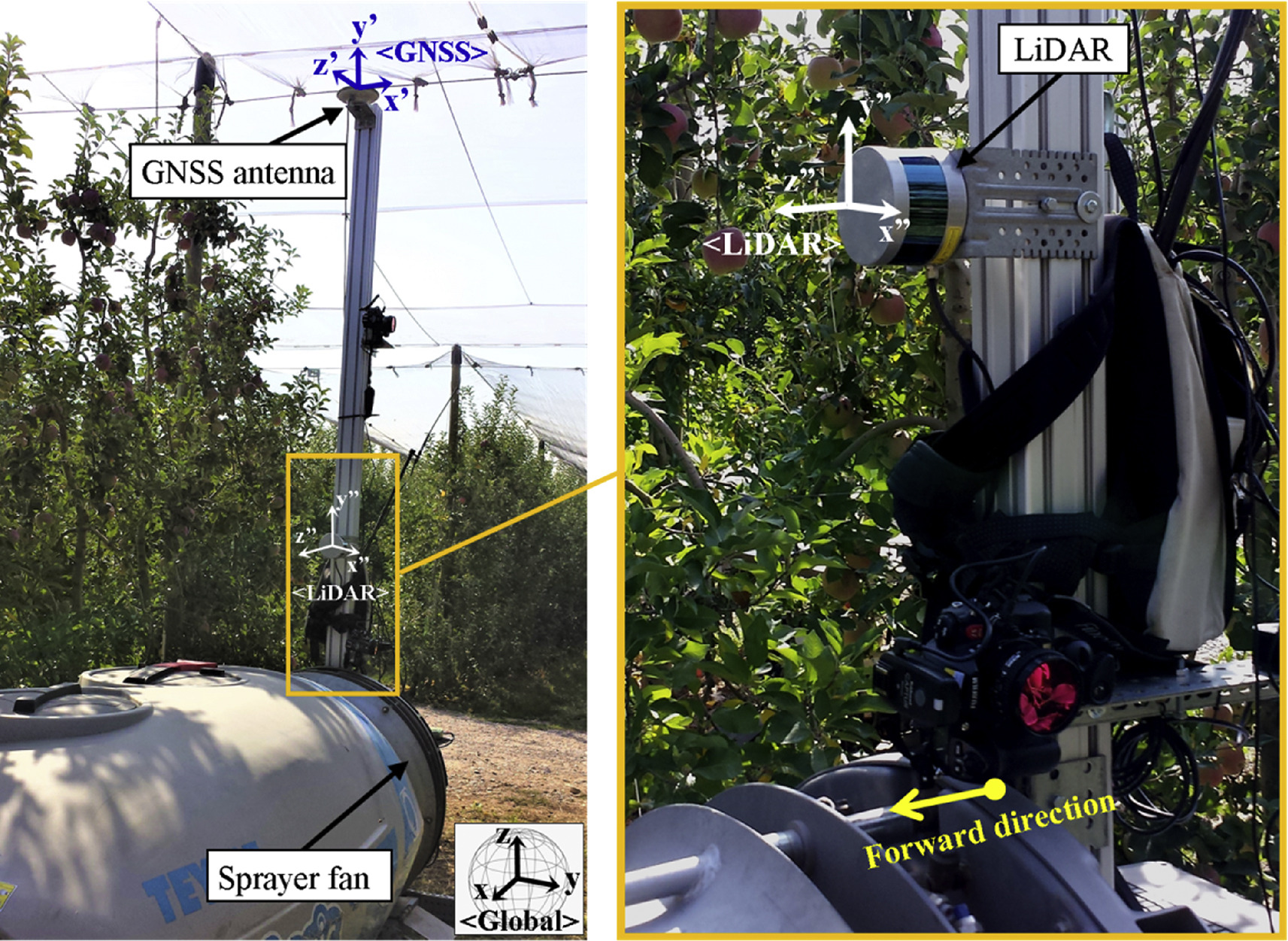
Authors: Gené-Mola, J.; Gregorio, E., Auat, F., Guevara, J., Llorens, J., Sanz-Cortiella, R., Escolà, A., Rosell-Polo, J.R., 2020. LFuji-air dataset: Annotated 3D LiDAR point clouds of Fuji apple trees for fruit detection scanned under different forced air flow conditions. Data in Brief 29, 105248. https://doi.org/10.1016/j.dib.2020.105248
Abstract: This article presents the LFuji-air dataset, which contains LiDAR based point clouds of 11 Fuji apples trees and the corresponding apples location ground truth. A mobile terrestrial laser scanner (MTLS) comprised of a LiDAR sensor and a real-time kinematics global navigation satellite system was used to acquire the data. The MTLS was mounted on an air-assisted sprayer used to generate different air flow conditions. A total of 8 scans per tree were performed, including scans from different LiDAR sensor positions (multi-view approach) and under different air flow conditions. These variability of the scanning conditions allows to use the LFuji-air dataset not only for training and testing new fruit detection algorithms, but also to study the usefulness of the multi-view approach and the application of forced air flow to reduce the number of fruit occlusions. The data provided in this article is related to the research article entitled “Fruit detection, yield prediction and canopy geometric characterization using LiDAR with forced air flow”
Fuji-SfM dataset: A collection of annotated images and point clouds for Fuji apple detection and location using structure-from-motion photogrammetry
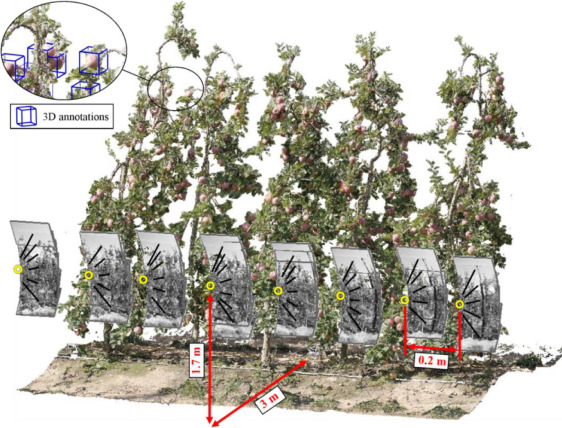
Authors: Gené-Mola, J., Sanz-Cortiella, R., Rosell-Polo, J.R., Morros, J.R., Ruiz-Hidalgo, J., Vilaplana, V., Gregorioa, E.,2020. Fuji-SfM dataset: A collection of annotated images and point clouds for Fuji apple detection and location using structure-from-motion photogrammetry. Data in Brief 30, 105591. https://doi.org/10.1016/j.dib.2020.105591
Abstract: The present dataset contains colour images acquired in a commercial Fuji apple orchard (Malus domestica Borkh. cv. Fuji) to reconstruct the 3D model of 11 trees by using structure-from-motion (SfM) photogrammetry. The data provided in this article is related to the research article entitled “Fruit detection and 3D location using instance segmentation neural networks and structure-from-motion photogrammetry” [1]. The Fuji-SfM dataset includes: (1) a set of 288 colour images and the corresponding annotations (apples segmentation masks) for training instance segmentation neural networks such as Mask-RCNN; (2) a set of 582 images defining a motion sequence of the scene which was used to generate the 3D model of 11 Fuji apple trees containing 1455 apples by using SfM; (3) the 3D point cloud of the scanned scene with the corresponding apple positions ground truth in global coordinates. With that, this is the first dataset for fruit detection containing images acquired in a motion sequence to build the 3D model of the scanned trees with SfM and including the corresponding 2D and 3D apple location annotations. This data allows the development, training, and test of fruit detection algorithms either based on RGB images, on coloured point clouds or on the combination of both types
KEvOr dataset: Kinect Evaluation in Orchard conditions
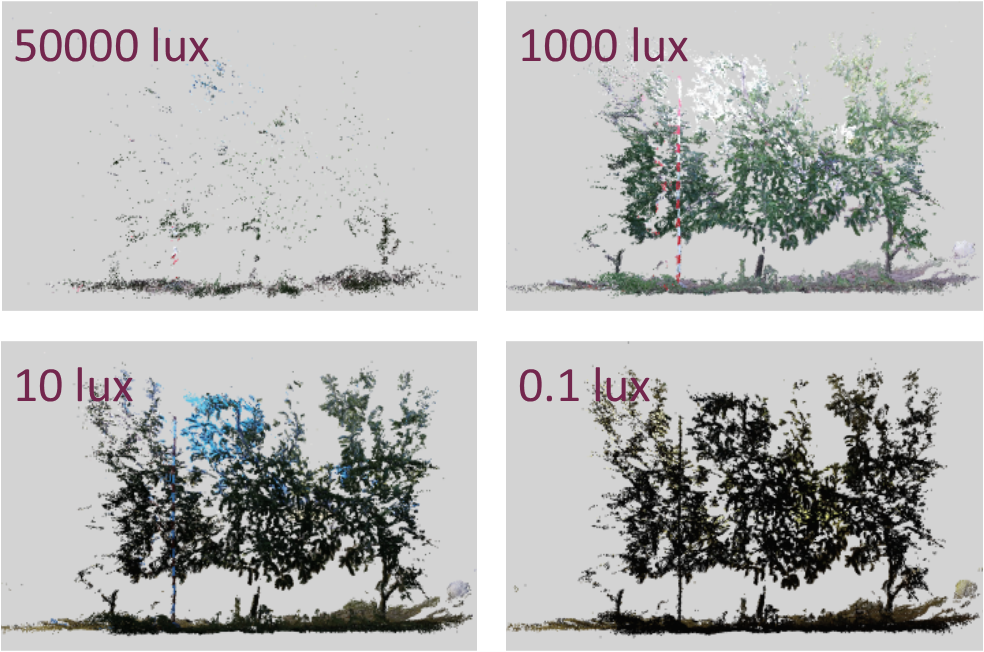
Authors: Gené-Mola, J., Llorens, J., Rosell-Polo, J.R., Gregorio, E., Arnó, J., Solanelles, F., Martínez-Casasnovas, J.A., Escolà, A., 2020. KEvOr dataset. Zenodo, https://doi.org/10.5281/zenodo.4286460
Abstract: The Kinect Evaluation in Orchard conditions (KEvOr) dataset is comprised of a set of RGB-D captures carried out with the Microsoft Kinect v2 to evaluate the performance of this sensor at different lighting conditions in agricultural orchards and from different distances to the measured target. Three Microsoft Kinect v2 sensors (K2S1, K2S2 and K2S3) were used to scan Fuji apple trees along the afternoon and evening, from the higher sun illuminance (55000 lux) until achieving dark conditions (0.1 lux), obtaining a total of 252 captures: 28 lighting conditions * 3 sensors * 3 repetitions. The data provided for each capture is: the acquired point cloud, illuminance level at the center of the measured scene, and wind speed. The sensors where placed as follows: K2S1: Oriented to the north, measuring the row of trees side under direct sunlight. This sensor was placed at 2.5 m from the measured target; K2S2: Oriented to the north, measuring the row of trees side under direct sunlight. This sensor was placed at 1.5 m from the measured target; K2S3 Oriented to the south, measuring the row of trees side under indirect sunlight. This sensor was placed at 2.5 m from the measured target; The dataset also includes two additional registered captures measuring the same scene from 1.5 m and from 2.5 m.
PFuji-Size dataset: A collection of images and photogrammetry-derived 3D point clouds with ground truth annotations for Fuji apple detection and size estimation in field conditions
Authors: Gené-Mola, J.,Sanz-Cortiella, R., Rosell-Polo, J.R., Escolà, A., Gregorio, E., 2021. PFuji-Size dataset: A collection of images and photogrammetry-derived 3D point clouds with ground truth annotations for Fuji apple detection and size estimation in field conditions. Data in Brief 39, 107629. https://doi.org/10.1016/j.dib.2021.107629
The PFuji-Size dataset is comprised of a collection of 3D point clouds of Fuji apple trees (Malus domestica Borkh. cv. Fuji) scanned at different maturity stages and annotated for fruit detection and size estimation. Structure-from-motion and multi-view stereo techniques were used to generate the 3D point clouds of 6 complete Fuji apple trees containing a total of 615 apples. The resulting point clouds were 3D segmented by identifying the 3D points corresponding to each apple (3D instance segmentation), obtaining a single point cloud for each apple. All segmented apples were labelled with ground truth diameter annotations. Since the data was acquired in field conditions and at different maturity stages, the set includes different fruit diameters -from 26.9 mm to 94.8 mm- and different fruit occlusion percentages due to foliage. In addition, 25 apples were photographed 360° in laboratory conditions, obtaining high resolution 3D point clouds of this sub-set. To the best of the authors’ knowledge, this is the first publicly available dataset for apple size estimation in field conditions. This dataset was used to evaluate different fruit size estimation methods in the research article titled “In-field apple size estimation using photogrammetry-derived 3D point clouds: comparison of 4 different methods considering fruit occlusion”.
A deep learning method to detect and measure partially occluded apples based on simultaneous modal and amodal instance segmentation
Authors: Gené-Mola, J., Ferrer-Ferrer, M., Gregorio, E., Blok, P.M., Hemming, J., Morros, J.R., Rosell-Polo, J.R., Vilaplana, V., Ruiz-Hidalgo, J. 2023. Looking behind occlusions: A study on amodal segmentation for robust on-tree apple fruit size estimation. Computers and Electronics in Agriculture, 209, 107854. https://doi.org/10.1016/j.compag.2023.107854
We provide a deep-learning method to better estimate the size of partially occluded apples. The method is based on ORCNN (https://github.com/waiyulam/ORCNN) and sizecnn (https://git.wur.nl/blok012/sizecnn), which extended Mask R-CNN network to simultaneously perform modal and amodal instance segmentation. The amodal mask is used to estimate the fruit diameter in pixels, while the modal mask is used to measure in the depth map the distance between the detected fruit and the camera and calculate the fruit diameter in mm by applying the pinhole camera model.
AK_ACQS Azure Kinect Acquisition System
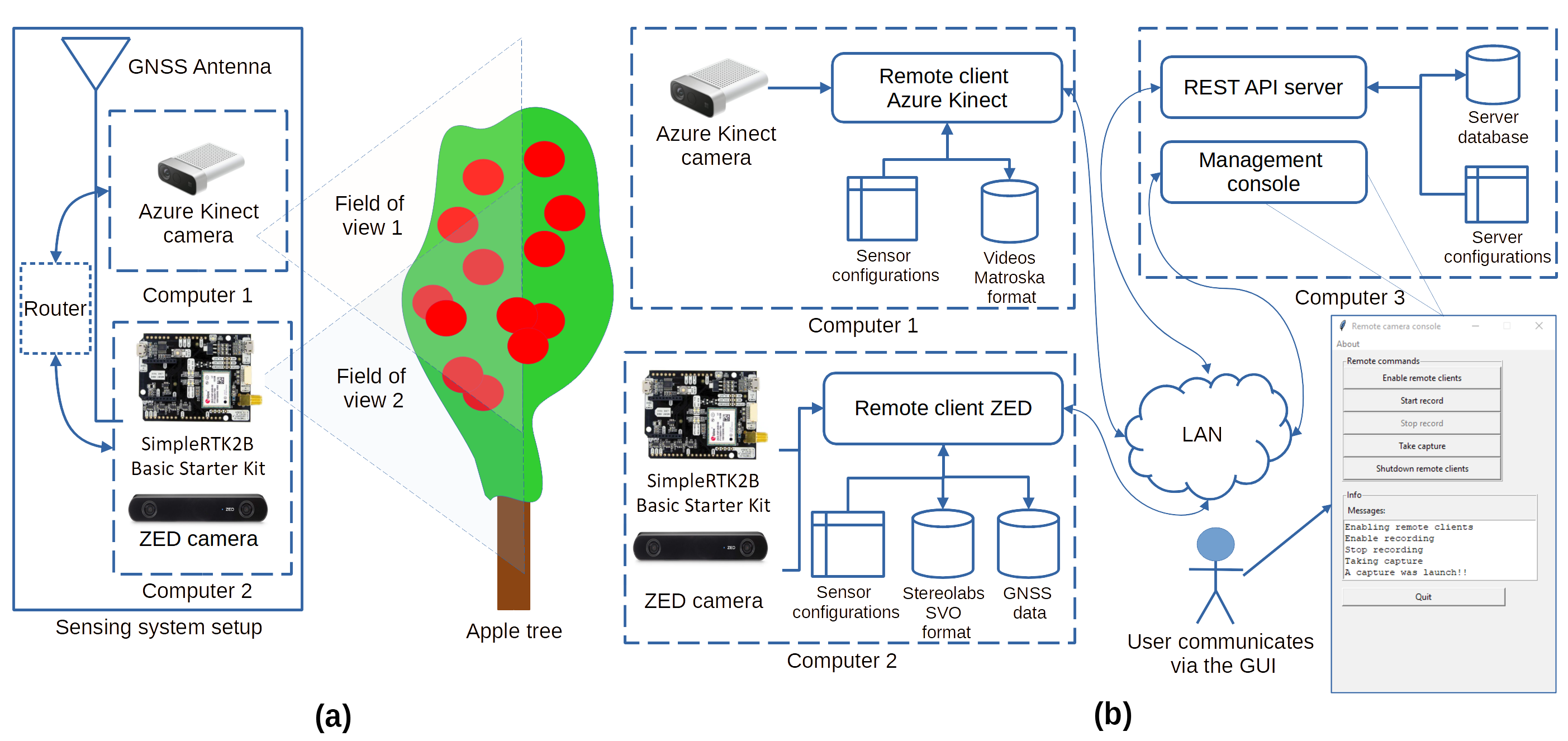
AKFruitData: AK_SM_RECORDER - Azure Kinect SM Recorder
Miranda, J.C., Gené Mola, J., Arnó, J., Gregorio, E., 2022. AKFruitData: AK_SM_RECORDER - Azure Kinect SM Recorder. https://github.com/GRAP-UdL-AT/ak_sm_recorder
A simple GUI recorder based on Python to manage Azure Kinect camera devices in a standalone mode. Visit the project site at https://pypi.org/project/ak-sm-recorder/
This work is a result of the RTI2018-094222-B-I00 project (PAgFRUIT) granted by MCIN/AEI and by the European Regional Development Fund (ERDF). This work was also supported by the Secretaria d’Universitats i Recerca del Departament d’Empresa i Coneixement de la Generalitat de Catalunya under Grant 2017-SGR-646. The Secretariat of Universities and Research of the Department of Business and Knowledge of the Generalitat de Catalunya and Fons Social Europeu (FSE) are also thanked for financing Juan Carlos Miranda’s pre-doctoral fellowship (2020 FI_B 00586).
AKFruitData: AK_FRAEX - Azure Kinect Frame Extractor
Miranda, J.C., Gené Mola, J., Arnó, J., Gregorio, E., 2022. AKFruitData: AK_FRAEX - Azure Kinect Frame Extractor.
Python-based GUI tool to extract frames from video files produced with Azure Kinect cameras. Visit the project site at https://pypi.org/project/ak-frame-extractor/ and check the source code at https://github.com/GRAP-UdL-AT/ak_frame_extractor/
This work is a result of the RTI2018-094222-B-I00 project (PAgFRUIT) granted by MCIN/AEI and by the European Regional Development Fund (ERDF). This work was also supported by the Secretaria d’Universitats i Recerca del Departament d’Empresa i Coneixement de la Generalitat de Catalunya under Grant 2017-SGR-646. The Secretariat of Universities and Research of the Department of Business and Knowledge of the Generalitat de Catalunya and Fons Social Europeu (FSE) are also thanked for financing Juan Carlos Miranda’s pre-doctoral fellowship (2020 FI_B 00586). The work of Jordi Gené-Mola was supported by the Spanish Ministry of Universities through a Margarita Salas postdoctoral grant funded by the European Union - NextGenerationEU. The authors would also like to thank the Institut de Recerca i Tecnologia Agroalimentàries (IRTA) for allowing the use of their experimental fields, and in particular Dr. Luís Asín and Dr. Jaume Lordán who have contributed to the success of this work.
AmodalAppleSize_RGB-D dataset: RGB-D images of apple trees annotated with modal and amodal segmentation masks for fruit detection, visibility and size estimation
Gené-Mola J., Ferrer-Ferrer M., Hemming J., van Dalfsen P., de Hoog D., Sanz-Cortiella R., Rosell-Polo J.R., Morros, J.R., Vilaplana V., Ruiz-Hidalgo J., Gregorio E., 2024. AmodalAppleSize_RGB-D dataset: RGB-D images of apple trees annotated with modal and amodal segmentation masks for fruit detection, visibility and size estimation. Data in Brief 52, 2024, 110000. https://doi.org/10.1016/j.dib.2023.110000.
The present dataset comprises a collection of RGB-D apple tree images that can be used to train and test computer vision-based fruit detection and sizing methods. This dataset encompasses two distinct sets of data obtained from a Fuji and an Elstar apple orchards. The Fuji apple orchard sub-set consists of 3925 RGB-D images containing a total of 15,335 apples annotated with both modal and amodal apple segmentation masks. Modal masks denote the visible portions of the apples, whereas amodal masks encompass both visible and occluded apple regions. Notably, this dataset is the first public resource to incorporate on-tree fruit amodal masks. This pioneering inclusion addresses a critical gap in existing datasets, enabling the development of robust automatic fruit sizing methods and accurate fruit visibility estimation, particularly in the presence of partial occlusions. Besides the fruit segmentation masks, the dataset also includes the fruit size (calliper) ground truth for each annotated apple. The second sub-set comprises 2731 RGB-D images capturing five Elstar apple trees at four distinct growth stages. This sub-set includes mean diameter information for each tree at every growth stage and serves as a valuable resource for evaluating fruit sizing methods trained with the first sub-set. The present data was employed in the research paper titled “Looking behind occlusions: a study on amodal segmentation for robust on-tree apple fruit size estimation”.